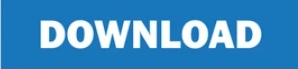
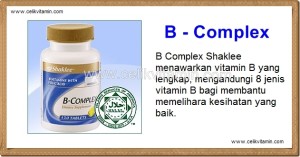
CC/PBSA, 58 FoldX, 38 Rosetta, 59 CUPSAT, 60 SDM, 48 and PoPMuSiC 61), 44 and some are machine-learning methods trained on large data sets at the potential cost of less interpretable results (e.g. Some “mechanistic” structure-based models apply parametrized molecular mechanics force-fields or substitution frequencies in the context of the local environment (e.g. Examples of sequence-based methods, which are beyond the scope of this work, include SAAFEC-SEQ 57 and the sequence version of I-Mutant. 5, 56 Comparing this stability to that of the wild-type protein gives the change in fold stability (ΔΔG, typically in kcal/mol) caused by the mutation, which is the primary output of most computational models.
AUTOMUTE PROTEIN FREE
Protein stability is conveniently measured as the free energy of unfolding (ΔG f) from thermal or chemical denaturation experiments. 43- 51 Several recent reviews address this topic in detail.
AUTOMUTE PROTEIN FULL
32- 37 Accordingly, intense efforts are directed toward computer models that can predict how an introduced amino acid mutation affects the stability of a protein, 38, 39 or the free energy of protein–protein binding/interaction, 40- 42 either using the amino acid primary sequence or more commonly, as the focus of the present article, a full structure from the Protein Data Bank (PDB), as input. 21- 27Īccurate prediction of mutation-induced protein stability changes may lead to more proficient screening of new disease-causing variants especially for diseases where protein stability plays a role beyond specific activity (these two contributions are hard to separate) 28- 31 and rational engineering of robust industrial proteins. 4- 11 A particularly important topic is how amino acid substitutions change stability, as this phenomenon restricts natural evolution in trade-offs between protein stability and function 2, 12- 20 and may contribute to many diseases associated with loss of protein fold structure such as Alzheimer's disease and Creutzfelt-Jakob disease. 1- 3 The determinants of this thermodynamic stability have been an ongoing research topic since the beginning of protein science. The ability of a protein to function depends on a sufficiently stable folded state. The simple models provide advantage in terms of interpretability, use and future improvement, and are freely available on GitHub. the S sym data set) while still performing well for all data sets ( R ~ 0.46–0.54, MAE = 1.16–1.24 kcal/mol). SimBa-SYM, despite is simplicity, is essentially non-biased (vs.
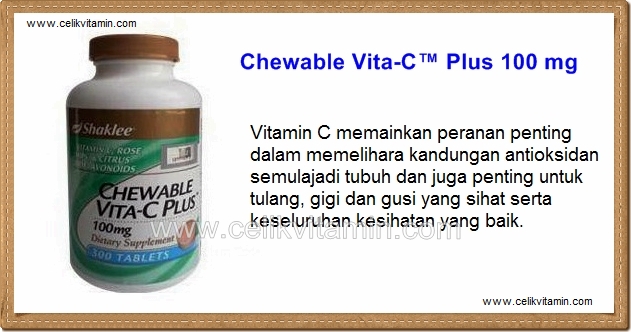
We developed two final models: SimBa-IB for typical natural mutations and SimBa-SYM for situations where stabilizing and destabilizing mutations occur to a similar extent.

Model structure and performance substantially depended on training data and even fitting method. The models were externally validated on three test data sets probing different pathologies and for internal consistency (symmetry and neutrality).
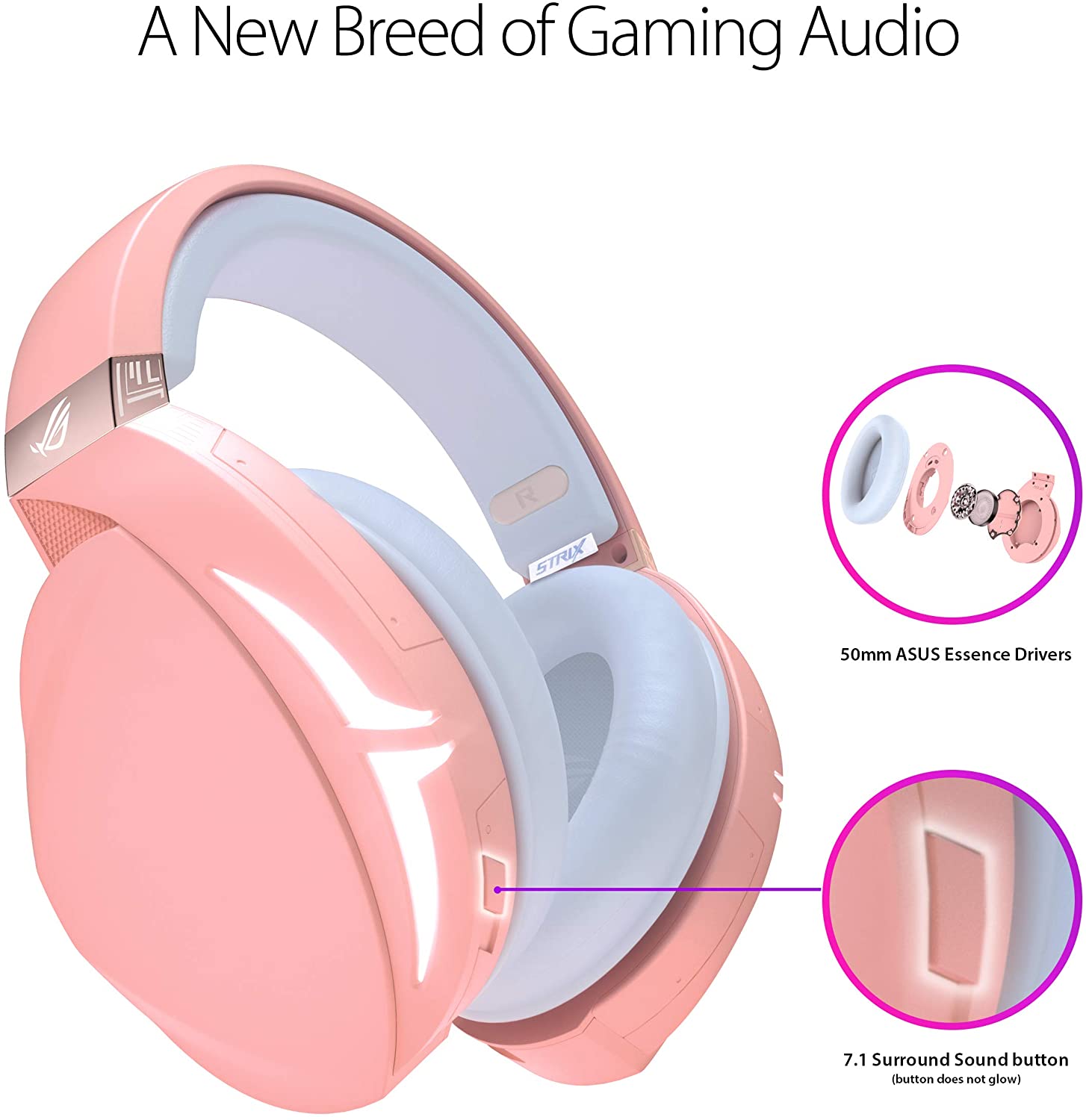
We investigated 45 linear regression models trained on data sets that account systematically for destabilization bias and mutation-type bias B M. Accurate prediction of protein stability changes upon mutation (ΔΔG) is increasingly important to evolution studies, protein engineering, and screening of disease-causing gene variants but is challenged by biases in training data.
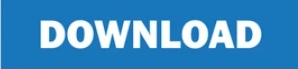